What’s Holding in up Widespread Data Science Adoption Banks?
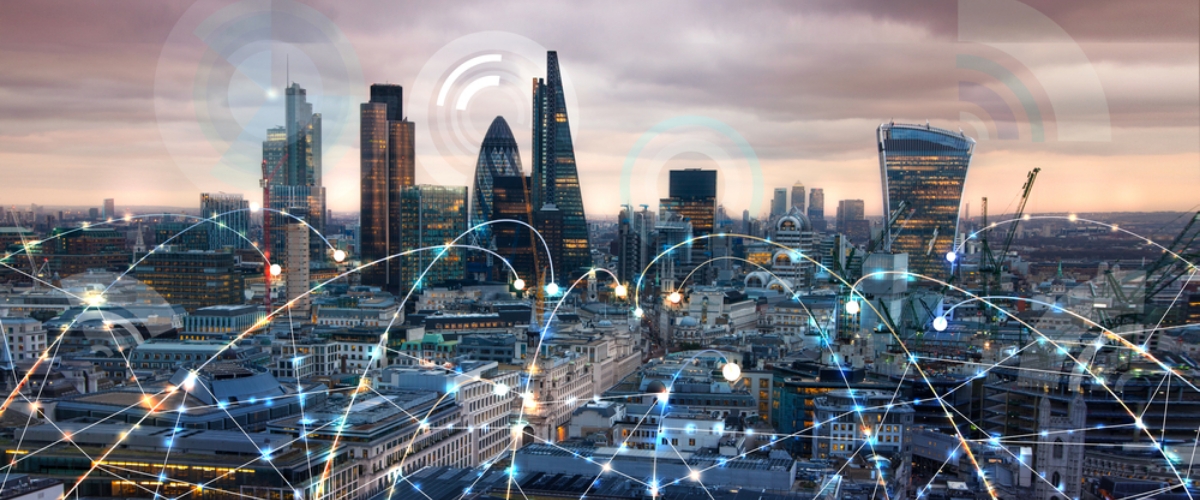
Banks have always had large reservoirs of customer data. The growing number of customer touchpoints is exponentially driving up the volume of data too. It is crucial to develop the capability to collect, interpret, and leverage it fast enough to drive service and product improvements and improve operational efficiencies.
Data science can help banks drive efficiencies and improve effectiveness, whether personalizing experiences, optimizing cross-sell offers, or making compliance more accessible and robust. Given the potential, it shouldn’t be surprising to see banks and financial institutions lean heavily towards data science to improve interactions and engage with their customers meaningfully. So, what is the reality of data science adoption?
Indeed, technology-led innovations such as blockchain, open banking, mobile banking, and AI and machine learning are now finding more use cases in this sector. AI chatbots, for example, have evolved and can now handle many interactions that humans once held. But, it’s fair to say the sector lags behind many others in adopting data science.
This then begs the question, if data science delivers such great value, then what is holding up its widespread adoption in the banking industry?
Skills Outstrip Supply
One of the most significant barriers to adopting data science is the growing skills gap in this line of work. Creating data science use cases and a data science roadmap for the bank, developing the right algorithms, and exploring the data to apply the right data and data sets become essential skills to drive these initiatives.
Data science is booming and is all set to create the circumstances to replace many legacy functions. However, while the number of roles needing data scientists is increasing, finding qualified data scientists to develop and run data science initiatives is challenging for banks across the globe.
It’s hard for banks to hire, engage, challenge, and retain data scientists who are often keen to seek roles that allow them wider and deeper exposure in this specialized area. This is why many banks partner with expert service providers like Ellicium Technologies to address their data science needs through innovative services and solutions models.
Infrastructure Challenges
Organizations need access to the right data science platforms and technologies to ensure that data science initiatives drive business outcomes. Data science platforms have specific infrastructure needs. Exploring vast data sets demands high computational capabilities as well. Something that the infrastructure of the organization has to support.
The banking sector, however, is still mainly working with legacy systems that make it hard to cope with growing data workloads or cater to the demands of modern analytics tools. Collecting, storing, securing, and analyzing massive data volumes with an outdated infrastructure can not only be counterproductive but can put the entire system’s stability at risk.
Enhancing processing capabilities, re-building their systems to manage the growing volumes of data, and enabling new-age technology applications and algorithms become essential for developing data science capabilities. Of course, this isn’t an easy task.
However, given the high stakes in the banking sector, it becomes essential to build robust on-premise or cloud infrastructures that fulfill the computational and data needs and allow banks to harness the power of data science.
Navigating the Algorithm Complexities
Data scientists spend most of their time in high-level languages such as Python/R/SQL. While several data science platforms offer ready-to-use, heavily optimized libraries, developing the right algorithms for data science according to the use case can need specialized customization. From putting together the right datasets to creating algorithms and integrating the analytics findings into business processes is a complex process that most banks seem to need help to address meaningfully.
Navigating the algorithmic complexity and understanding how well code scales with data, identifying all the elements that go into algorithmic performance, and clearly understanding and managing algorithmic complexities are essential data science elements. Assessing which would be the right language to fulfill algorithmic needs while fulfilling the needs of the domain and business case also become important consideration points that are challenging for most banks.
Apart, rapid production deployment of algorithms and ensuring regular tuning and maintenance of these algorithms also involve some heavy lifting. Provisioning the right skillsets, building up experience, and training resources remain pain points for banks.
The “Proof of concepts” Pudding
The banking sector has been more cautious in its technology appetite, given the strict regulatory and governance landscape and the high-security needs of this sector. As such, establishing the right proof of concepts employing the right technologies for data science solutions becomes an inevitable need before embarking on the data science journey.
The absence or the inability to deliver these proof of concepts can damage or impede any data science initiative as it becomes difficult to identify the RoI and the tangible benefits of using this technology. The proof of the pudding, after all, is in the eating. An associated challenge is the inability to scale beyond the POC because of poor planning. Many banks stay stuck in “POC Purgatory” because they are unable to define the success criteria for the pilot projects or chart roadmaps for the way forward should the pilot succeed. Data science pilots at such banks remain nothing more than costly curiosities or expensive experiments.
While developing in-house data science capabilities is ideal, banks have to ensure that they develop these capabilities fast, given the accelerating pace of change. As the value of data keeps depleting and competition increases, only those who can leverage data science fast can stay ahead of the curve by creating compelling competitive differentiation. If an in-house data science team needs to cut, identifying the right technology partners to assist the data science journey becomes crucial for organizational and data science success. May we suggest talking to us as a first step on your data science journey?