The Critical Importance of Building an Effective Lakehouse Solution
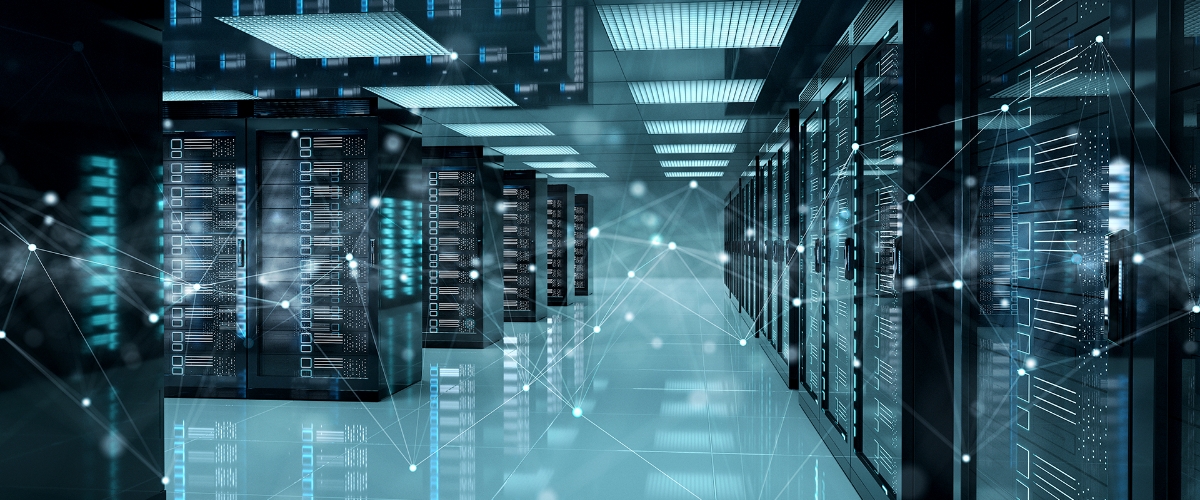
As data becomes the new fuel, companies across sizes and sectors have begun collecting large amounts of data from all sources possible – in the hope of unearthing insights previously unheard of. At the same time, data powers every business decision today; storing this snowballing amount of data hasn’t been easy.
While data warehouses and data lakes serve as ideal repositories for storing raw data in different formats, both rely on other processes for capturing data. Each has its own boundaries around specific transactions, such as data quality controls, batch processing, etc.
With data complexity only increasing yearly, there is a pressing need for a centralized, flexible, and high-performance system that can handle diverse data requirements such as SQL analytics, real-time monitoring, data science, and machine learning.
What is a Lakehouse?
Advances in data analytics mechanisms and innovations in AI have led to more complex and multiple data storage, analysis and governance systems. A standalone data warehouse must be optimized for such complexities, compelling data architects to rely on multiple data lakes, warehouses, and other specialized systems to process modern-day data.
A lakehouse is a new data storage and management approach that combines the best of both worlds – data lakes and data warehouses. It offers a new and open architecture enabled by better system design by merging ease of access and support for enterprise analytics capabilities found in data warehouses with the relatively low cost and flexibility of data lakes.
What Value Can Enterprises Derive from it?
Lakehouses eliminate the need for organizations to use many systems for data storage and management, thus removing unnecessary management overhead. For instance, the transactional metadata layer allows architects to apply data management techniques on top of raw, low-cost storage while constantly caching, indexing, and optimizing data.
They further allow organizations to leverage improvements in data architectures, data processing mechanisms, and metadata management to capture all data to a common platform and efficiently share it for machine learning and BI applications.
Using lakehouses, organizations can:
- Enable concurrent reading and writing of data across different data pipelines using SQL
- Help data architects use multiple BI and Reporting tools directly on source data, thus reducing latency, improving recency, and lowering costs.
- Decouple storage from computation to allow multiple concurrent users and support larger data sizes.
- Ensure open and standardized storage formats, avoid vendor lock-in, and keep a range of modern APIs.
- Power diverse workloads, including data science, machine learning, and SQL and analytics
Key Challenge in Lakehouse Adoption
Although lakehouses can simplify the entire approach to modern-day data engineering by providing a centralized repository for all types of data and applications, they take work to implement. As a relatively new architecture, many organizations still need to learn about the technology or know how best to harness its benefits.
Likely, organizations may need to upskill their current data engineering team or invest in an entirely new one with the required skillsets to run complex metadata management layers that eventually organize/optimize data on the fly. At the same time, businesses may also need to onboard competent data architects and scientists who can optimize data storage and representation in response to specific AI or ML workloads.
How can an Expert Partner Help?
Bringing years of experience and expertise in complex data engineering and management, a partner like Ellicium can help build a robust lakehouse and ensure that data available to decision-makers is always complete and accurate and caters to all data types and formats.
By allowing storage of all structured and unstructured data at large scales in a lakehouse, Ellicium can enable complex processing and run different types of analytics for timely and accurate decision-making.
Ellicium can help:
- Provide advisory services and a roadmap on how to build a lakehouse.
- Design a tailored lakehouse, keeping business goals, and end-user reporting needs in mind.
- Set up an efficient lakehouse using Big Data and cloud technologies like Hadoop, AWS, Azure, etc.
- Efficiently extract unstructured data from external sources and parse it using.
- NLP techniques and machine learning algorithms
- Build the right frameworks to ingest and analyze real-time data from systems, IoT devices, and logs.
- Enable effective data modelling based on industry standards and business-specific models and requirements.
- Deploy ETL tools like Talend, Informatica, etc., and schedulers for integrating, orchestrating, and scheduling the lakehouse processes.
- Implement the required level of security and governance while also adhering to industry standards, best practices, and benchmarks.
- Enable native support for AI and machine learning algorithms while optimizing data for fast query performance.
Lakehouses radically simplify enterprise data management without toggling between multiple systems by offering improved architecture, necessary data versioning, governance, and security controls. They allow structured/unstructured data analysis via modern AI and BI tools to keep pace with modern-day analytics requirements.
Connect with us to learn more.